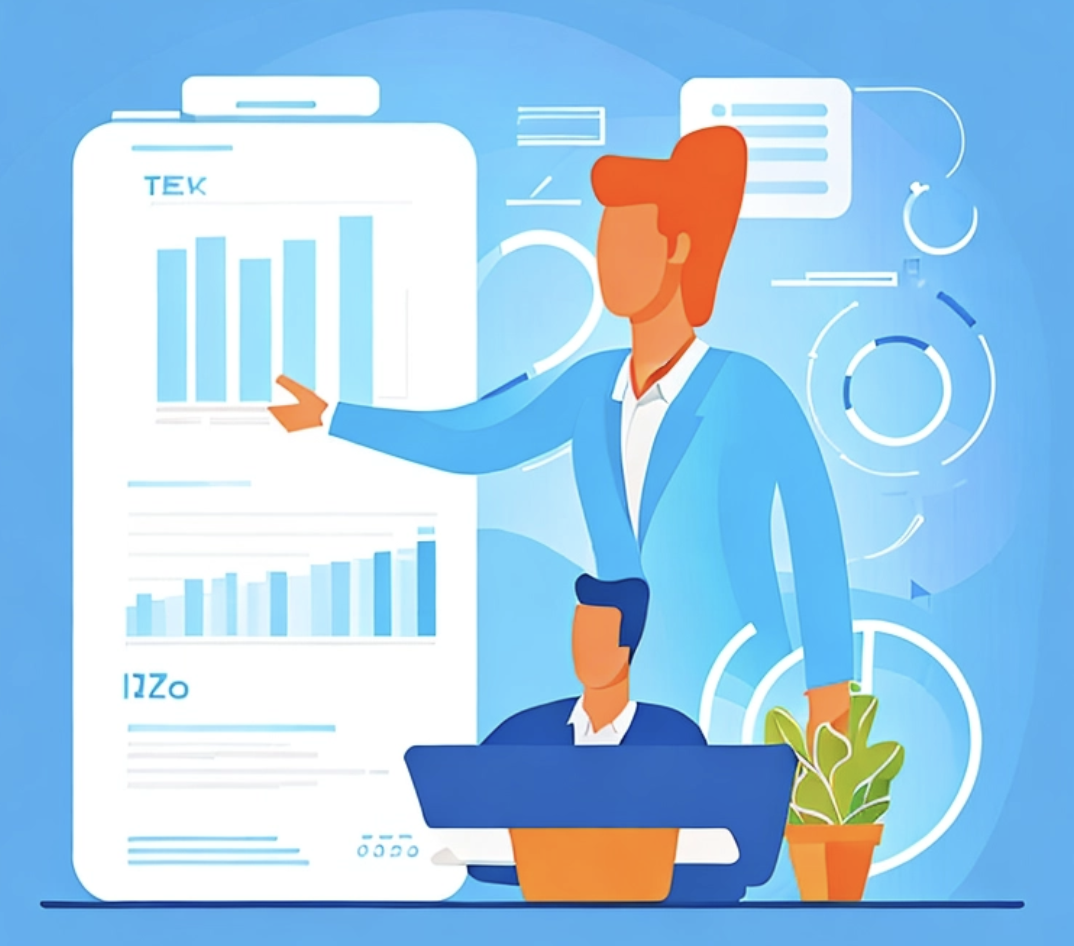
A vision of AI models
Discover how AI models improve mobile games and apps, focusing on user behavior and process optimization. Assetario aims to offer cost-effective solutions by leveraging past experiences, ultimately partnering with internal teams for seamless integration and superior performance.
Introduction
In this blog post, I'd like to discuss how AI models are being deployed across consumer businesses and apps. Consumer businesses are continually striving to enhance their user experience, understand their users better, and improve their internal processes to be more efficient. The consumer market is a significant competition for each user's time and money, so there is obvious pressure to improve every month.
As a result, each consumer app begins employing data analysts and data scientists early in the growth process.
At Assetario, we aim to identify use cases that can be generalized to multiple businesses, where the knowledge acquired from deploying at one customer can be directly applied to others. This puts us in a unique position, enabling us to deliver models that will achieve good performance metrics faster than a model developed internally (i.e., in "isolation"), and therefore, it will eventually be cheaper.
Building and deploying a model
Once a use case is identified, building the actual model itself is typically not particularly difficult.
To deploy a model internally, you first go through a standard data science process involving, in a general sense, data exploration and model development stages. After that, if the offline metrics and latency requirements are met, the model is approved for production.
However, deploying a model is certainly not the end of the story. If anything, it is just the beginning. Models are deployed "into reality," and reality changes. The behavior of your users might change over time, the distributions of the datasets used during the offline training stage might not match those observed after deployment, and we might encounter a number of edge cases that were initially not taken into account, among other factors.
Monitoring model performance and taking actions as performance degrades is an area often overlooked but perhaps the most crucial aspect to consider when deploying ML solutions.
Researching a model
At this point, we would like to emphasize the value that Assetario brings to the industry.
As mentioned earlier, we aim to build solutions that are easily deployed and perform well in a short period of time. This, of course, directly implies that our solutions are ultimately more cost-effective, as they generate the desired revenue shortly after deployment.
We achieve this through what we could refer to as "aggregated knowledge." At the time of writing this post, we have deployed on tens of enterprise clients. We have encountered and addressed a variety of technological setups and intellectual challenges. This places us in a unique position where, unless a use case is highly nuanced, it is likely that we have a solution ready to be used from our repertoire.
Conclusion
At Assetario, we aim to assist companies in building successful apps. Our value proposition relies on the fact that we have encountered a vast array of scenarios, resulting from deploying our solution for dozens of different customers. This extensive experience has enabled us to develop a suite of algorithms that can adapt to nearly any need our customers might have. With that in mind, our ultimate goal is to provide a service that seamlessly integrates with our customers' systems and outperforms models developed internally, all while doing so in a faster timeframe than it would take to develop those models.
It's important to note that Assetario currently specializes in mobile app monetization personalization. However, the underlying algorithms have the potential to be applied to a wide variety of use cases. Exploring these potential use cases would be straightforward once the data has been ingested and integrated into our systems.
Having said all of the above, we would like to emphasize that it is not our intention to replace internal teams, but rather to complement them and build trusted partnerships. From both a technical and business perspective, we possess a broad understanding of the industry, knowledge that can certainly be leveraged to help our customers make better decisions.